Machine learning is already an integral part of life. You can find it in various fields. It’s there in the household items you have at home. And it’s also there in the healthcare system.
The most basic example of machine learning is a computerized system to catalog patients’ data. But machine learning can do a lot more than cataloging work. Utilizing AI can massively improve the system for diagnosing and taking the necessary action.
The potential of utilizing machine learning
Utilizing AI in healthcare means there will be more projects covering more solutions in the future. Even something as simple as registration can benefit from assigning a specific algorithm. It will cut the time required to diagnose an issue and handle the patient accordingly.
A centralized healthcare registration system will help health practitioners across the country track their patients’ previous medications. They can tell if someone can take antibiotics or not. They also don’t have to guess if the patient has an underlying health problem that may not fit with the current medication.
The challenges it faces
One of the most prominent challenges of machine learning is the minimum information on what it can do. Most people conflate AI with everything robotic. While it is true up to a point, machine learning has so much potential that
Another challenge is the ethical accountability of leaving a machine to decide on a human’s life. Even though human health practitioners have flaws and make errors in their judgments, they work with a sense of compassion to support their moral compass. Those are two things that an AI lacks.
Even though the machine will stay precise in the assessment and decision. But it only is as good as the data and samples it has. In some cases, having data alone are not enough. Most doctors will look for a second or even third opinion to support their initial diagnosis. While a machine will only compile the data and build a diagnosis based on the information.
AI is also susceptible to cyber-attacks and other digital-world crimes. A hospital in Osaka, Japan had a ransomware attack for a couple of days and forced them to return to manual work. This attack affected up to 1,000 patients and staff the hospital has.
Four Examples of Machine Learning in Healthcare System Practice
Here are examples of utilizing AI in healthcare system practice. It’s only a fraction of what machine learning can do to help humanity in the future. There are no limits on how and what the AI can do to help the healthcare system.
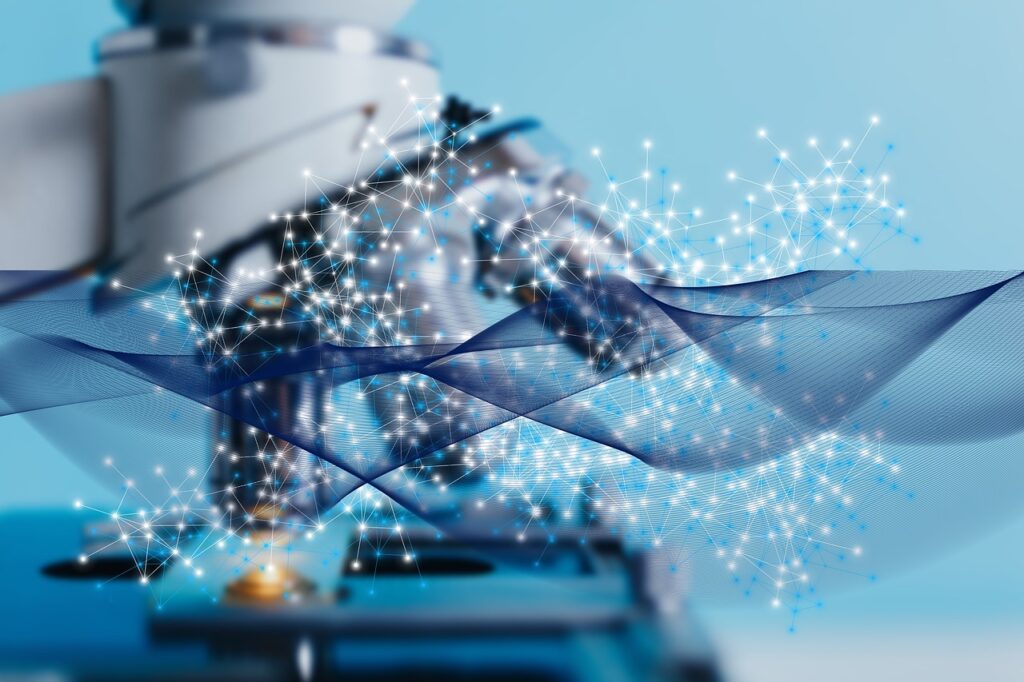
- Forecasting drug demands
Most drug companies are already using AI-based algorithms to forecast drug demands. AI helps them on multiple fronts. In addition, know when to adjust the production level. They also can predict the time allocation to improve their products.
The forecast will help the R&D team improve the drugs’ efficacy. It also helps the marketing team to phase out the old drugs and replace them with more effective ones. The public can benefit from the new drugs with higher efficacy. They also can rest assured knowing no bad or obsolete drugs are still in circulation.
- Early prediction of diabetes
Diabetes is of the top 5 killer diseases in the world. More than 400 million people worldwide have this condition. However, most are not aware until years later. Diabetes can also cause other problems such as blindness,
Unfortunately, most people are not aware of this condition until years later. Some lucky people got an early diagnosis and can take action quicker.
An example of this algorithm is using a DNA check. Diabetes is a hereditary condition that is passed through generations. Expecting parents with a history of diabetes in their family can get a blood test during the pregnancy to see the possibility of their children having one.
Numerous apps are already applying the algorithm for early diabetes detection. Users can input their health information like blood sugar level, skin tightness, and other information and let the app calculate the possibility of them having diabetes.
- Personalized healthcare system
Also Read: Unveiling Innovations in the Dental X-ray Tube Market 2023
Many hospitals are already implementing machine learning into their system and creating a personalized care systems. The hospital recognizes the issues and acts accordingly without burning its human resources.
Numerous success stories come from implementing AI in therapies. Health caretakers can see a massive improvement in their patient’s results. And machine learning also helps them adapt to new methods while still collecting data for future patients.
Machine learning also helps out-patients with following prescriptions and doing therapies at home. They can install an app that will connect their account and remind them every time they need to take their medications. And if there is an issue, the app will immediately contact emergency services.
- Reducing false results
The AI will give more accurate diagnoses as it compiles more data from various patients with different backgrounds. It means reducing the number of false diagnoses that lead to fatalities. But unfortunately, the number of cases diagnosed is not enough to say if the AI provides high accuracy.
For example, about 30% of breast cancer cases are false positives. But about 30% of breast cancer patients lost their lives to cancer due to a misdiagnosis. This is an issue that machine learning can work with. However, the number of people willing to get diagnosed is still slow.
Conclusion
Machine learning is more about automating most practices. But it’s more about using all possible methods toward a better and more efficient solution. And in healthcare system, AI covers multiple factors in supporting the field. They can use AI to work with patients, primarily on diagnosing diseases.
The irony is that AI relies on the data to improve, but it’s also one of the main challenges to building a good and responsive AI. There are still not enough data for the AI to diagnose and decide on the best action. Still, everyone can participate in improving AI by actively contributing to the data collection process.
Another issue with AI in healthcare system is accountability. There is still no regulation on holding machine learning responsible for any misdiagnoses leading to fatality.
Image by Darko Stojanovic from Pixabay